Attribution Detectives - Cracking the Code of Marketing Attribution
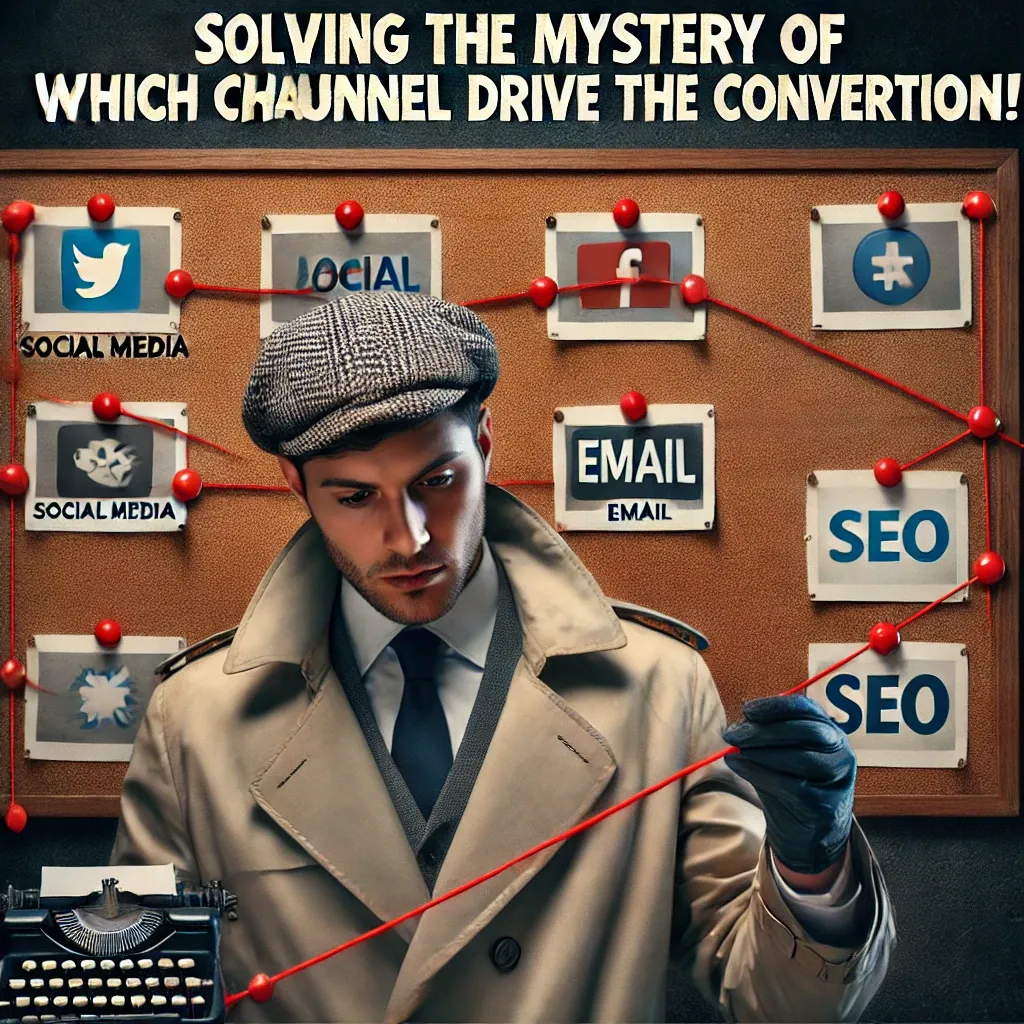
Imagine you’re the person in charge of marketing at a bicycle company. Your performance at the end of the year will be judged on one thing: how many incremental bikes you’ve sold.
Sounds straightforward, right? After all, most families buy at least one bike for their kids to learn on.
Unfortunately, it’s not that simple.
The market isn’t what it used to be—birth rates are down, and competition is fiercer than ever. To grow your sales, you need to invest in marketing.
During your first months on the job, you experiment. You launch social media campaigns on platforms like Meta and TikTok, aiming to catch the attention of new customers. You dabble in paid search ads, targeting people actively searching for bikes. You even sponsor local gyms, figuring active folks might want to take their fitness outdoors.
Before long, sales begin to climb. But here’s the catch: you have no idea which of these efforts is driving the results.
Is it the TikTok campaign that’s pushing customers to convert? Or are the search ads delivering the best bang for your buck? Maybe it’s a combination of both.
This is where marketing attribution comes in. It’s the key to understanding what’s truly working so you can double down on the strategies that move the needle.
The Attribution Detective: Using Frameworks to Find ROI
But how can you tell what is working (and what isn’t)?
Think of yourself as a detective, piecing together clues from every corner of your marketing ecosystem. Every click, view, and conversion leaves a digital fingerprint. Even traditional campaigns, like your gym sponsorships, leave behind clues—even if they’re harder to trace.
Your job is to follow these trails, identify patterns, and build a case that reveals which touchpoints play the biggest role in driving sales. To do so, marketers rely on attribution models.
What Are Attribution Models?
Attribution models are frameworks that help you understand how different marketing touchpoints contribute to a conversion. They use the clues left behind by a users digital activities to assign credit for conversions to marketing channels.
Imagine a customer’s journey as they encounter several marketing channels before finally purchasing a bike:
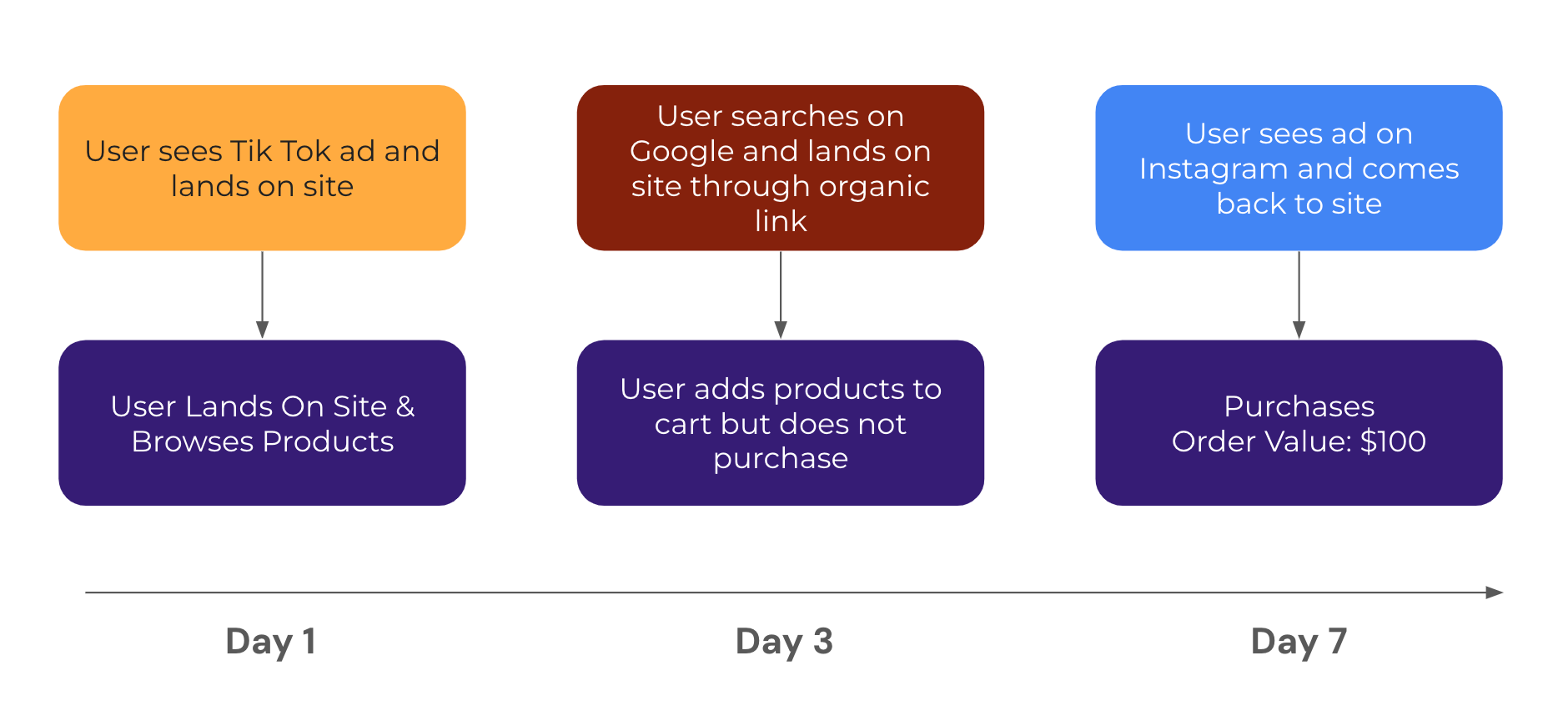
Should TikTok be credited with bringing the customer to your site first, or should Instagram be credited with nudging them to finalize their purchase?
The answer to which channel you attribute the revenue is very important. It will guide how you distribute your investment and impact the ROI of your future marketing efforts.
Rule-Based Attribution Models Explained
Before diving into rule-based attribution, remember that no single model is perfect. Each offers a unique perspective, shedding light on different aspects of your marketing mix.
By examining your customer journey through multiple lenses, you can discover which channels spark interest, nurture engagement, and close the deal.
Some of the classic rules-based attribution models are:
- Last Click Attribution: Assigns full credit to the final interaction before the sale. In our example, Instagram would receive $100 for the purchase.
- First Click Attribution: Credits the channel that introduced the customer to your brand. In this case, TikTok would get the $100.
- Linear Attribution: Distributes credit evenly across all interactions. TikTok, Google Organic Search, and Instagram would each receive $33.
- Time Decay Attribution: Weighs touchpoints more heavily as they get closer to the purchase. For example, TikTok might get $20, Google Organic Search $30, and Instagram $50.
- Position-Based (U-Shaped) Attribution: Prioritizes the first and last interactions while acknowledging the middle touchpoints. TikTok and Instagram might each get $40, with Google Organic Search receiving $20.
Pros and Cons of Each Model
Like a detective evaluating different suspects, each attribution model has strengths and weaknesses.
- Single-Touch Models (First Click, Last Click):
- Pros: Simple to use and easy to understand.
- Cons: Overly simplistic, often ignoring other channels that play critical roles in the customer journey.
- Multi-Touch Models (Linear, Time Decay, Position-Based):
- Pros: Provide a more comprehensive view by considering the entire customer journey.
- Cons: More complex and may require additional effort to interpret.
In the end, no single model holds the truth. They are just frameworks to help you make sense of the data. The best choice depends on your goals and the nature of your customer’s journey.
Data-Driven Attribution: A Smarter Approach
Up until now, we’ve discussed what’s known as rule-based attribution—models where specific guidelines, like “credit the first interaction” or “split credit evenly across all touchpoints,” are used to assign credit for conversions. These rules are straightforward and easy to apply, but they rely on static assumptions rather than adapting to your actual data.
Data-driven attribution takes a more advanced approach. Instead of following fixed rules, it leverages machine learning to analyze patterns in your data. This model dynamically determines which touchpoints have the most impact by continuously learning from customer behavior and updating its calculations.
While the underlying mechanics can be complex, a simple way to understand data-driven attribution is this: it assigns credit to each touchpoint based on how that interaction changes the probability of conversion. Essentially, it evaluates the user journeys of both converters and non-converters, builds a probabilistic model, and uses techniques like Shapley values to interpret the results.
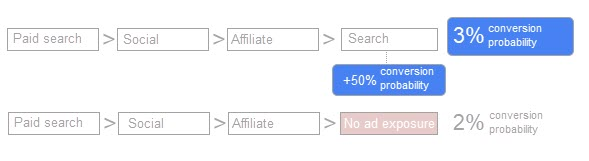
For example, if adding a search ad as the last touchpoint increases the probability of conversion by 50%, the model assigns credit proportionate to that impact. This allows a more precise understanding of how your marketing efforts work together to drive results without relying on rigid, predefined rules.
The Challenges of Attribution in a Privacy-Centered Age
Just like a detective who suddenly finds fewer witnesses willing to talk, marketers are facing a new era where data is becoming scarce.
Stricter privacy regulations such as GDPR, technological changes like the release of IOS 14 and subsequent versions, and users' adoption of ad blockers are quickly interrupting marketers' ability to track the entire funnel.
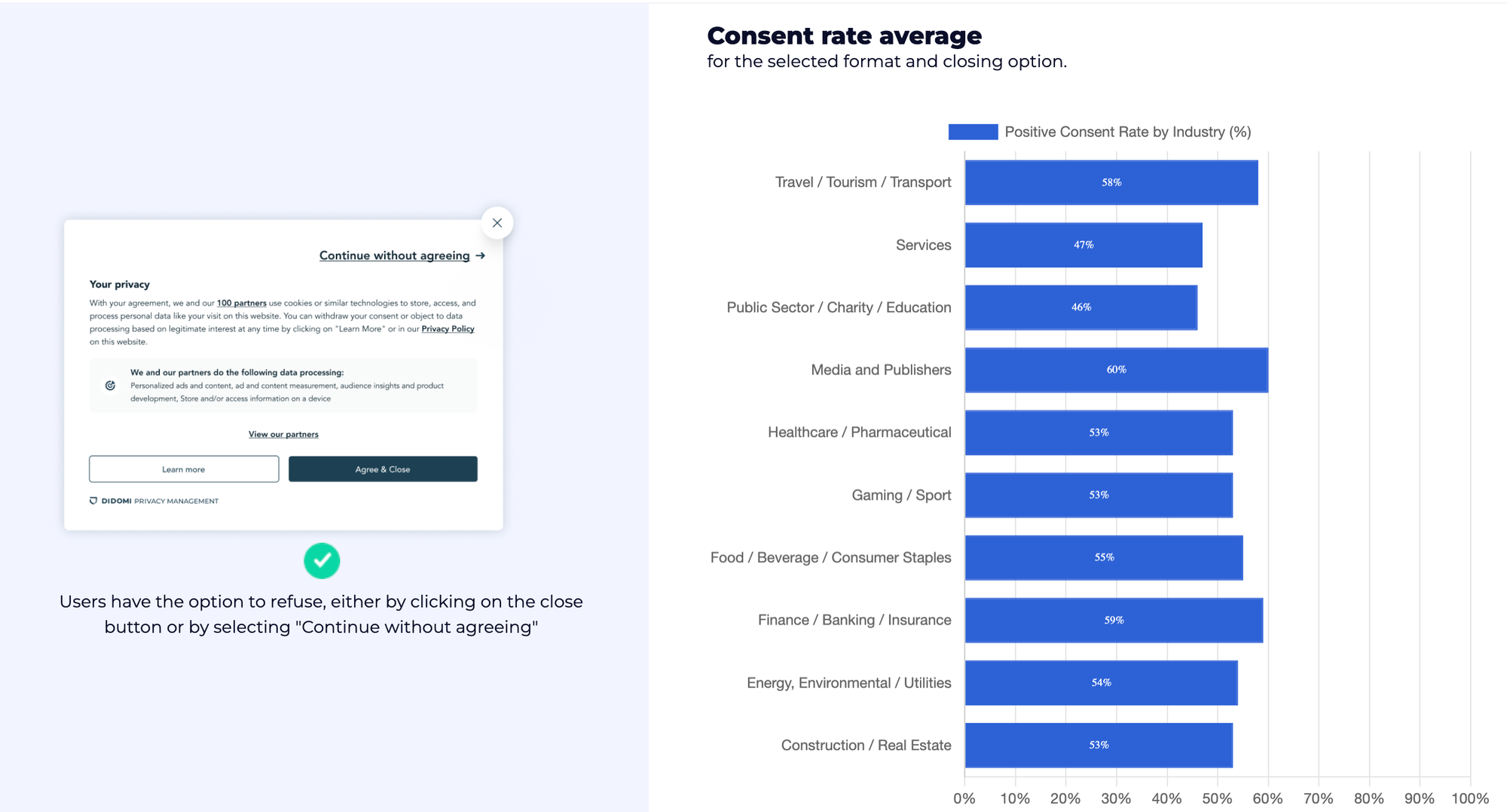
As data vanishes, our ability to attribute conversions to marketing actions diminishes, making our attribution models less trustworthy.
The Impact of Privacy Changes on Attribution
- Fragmented Data: Without the ability to track users across different sessions and websites, data becomes fragmented, making it harder to attribute conversions to specific campaigns or channels accurately.
- Shortened Attribution Windows: Cookies' reduced lifespan shrinks the time frame in which conversions can be attributed. This can lead to an incomplete picture of the customer journey, particularly for high-consideration purchases that require longer decision-making periods.
The lack of visibility into customer journeys forces marketers to rethink how they solve the attribution puzzle. Instead of focusing on granular user-level data, solutions that rely on aggregated data can help complete the picture.
Media Mixed Modeling and Regression-Based Attribution
Media Mix Modeling (MMM) and regression-based attribution are tools that look at the big picture instead of relying on granular data. They are actually quite ancient, but are experiencing a renaissance due to limits to user level tracking.
- Media Mix Modeling (MMM): This approach analyzes aggregated data, such as total ad spend, sales, and external factors like seasonality or market trends. By examining these relationships over time, MMM helps identify which marketing channels are driving results at a macro level. While it’s less detailed than user-level tracking, it provides valuable insights into overarching trends and helps allocate budgets effectively.
- Regression-Based Attribution: This technique uses statistical models to uncover the relationships between marketing activities and conversions. For example, if a spike in paid search ads correlates with an increase in sales, regression analysis can estimate how much of that lift is attributable to the ads. It’s a way of finding patterns in your data without needing to track individual users.
Both approaches offer a way forward in the privacy-centered era. They allow marketers to continue optimizing campaigns by shifting the focus from micro-level tracking to macro-level analysis.
In the next post in the series, we'll dive into these aggregated data techniques and create and run through a demo project using synthetic data.
Conclusion
Marketing attribution is no longer as straightforward as it once was. With privacy changes reshaping the landscape, marketers must adopt a detective’s mindset to uncover what truly drives conversions.
Whether you’re using rule-based models, exploring data-driven approaches, or turning to macro-level tools like Media Mix Modeling, the key is to keep experimenting and adapting.
Comments